![[BKEYWORD-0-3] What does latent function mean](https://image.slidesharecdn.com/socialinstitution-140123051312-phpapp01/95/social-institution-22-638.jpg?cb=1390454142)
DALL-E consists of two main components. A discrete autoencoder that learns to accurately represent images in a compressed latent space. And a transformer which learns the correlations between language and this discrete image representation. In part one of this series, we focused on understanding the autoencoder. While the exact techniques are a bit different, the core goal remains the same. Nonetheless, I will briefly explain the differences at the beginning of this post. The transformer is arguably the meat of DALL-E; it is what allows the model what does latent function mean generate new images that accurately fit with a given text prompt.
Beyond just creative and accurate designs, the transformer also seems to understand some common sense physics.
Navigation menu
Nobody knows exactly why transformers work so well, or even what they actually learn; there is no fundamental theory for deep learning that can explain all of this, these networks functiln sort of too big and complicated for us to fully understand currently. You train a big model with lots of data and follow a set of mostly empirically derived best practices and suddenly your model can generate images of Avocado chairs on command. No one can fully explain it; it just works.

Nonetheless there are some general intuitions that can help with understanding the capabilities and limitations of these types of models. Note: this blog post assumes that you have some knowledge of deep learning and Bayesian probability.
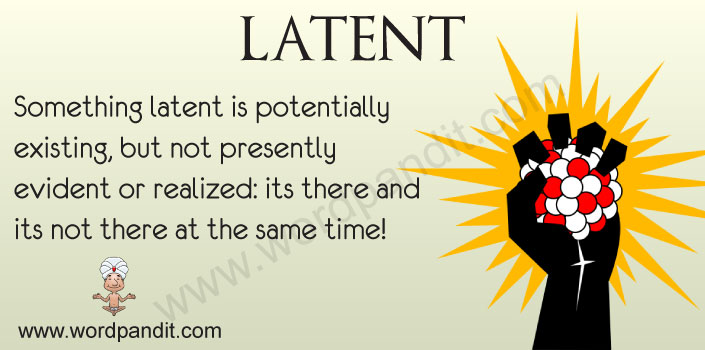
Before jumping into the transformer side of DALL-E, I want to briefly correct some of the assumptions made in part one. Now that the paper has finally been released, we can take a look at the details of their discrete autoencoder, which they call dVAE.
Representation Learning
Ultimately, the high level goal of dVAE is the same: it attempts to learn a discrete representation for images. Note: this section is somewhat disconnected from the rest of the blog post, so if you are more interested in the transformer stuff, feel free to skip to the next section. Recall that VQ-VAE learns a codebook; basically just an indexable lookup table for a finite set of learned vectors. The encoder network is then responsible for taking in an image and outputting a set of vectors, where each one is ideally close to some codebook vector.]
I suggest you to try to look in google.com, and you will find there all answers.
There was a mistake