Derivative of softmax Video
Derivative of Sigmoid and Softmax Explained Visually derivative of softmaxConsider, that: Derivative of softmax
Epic of gilgamesh analysis essay | 287 |
Stem cells quotes | In the context of artificial neural networks, the rectifier or ReLU activation function is an activation function defined as the positive part of its argument: = + = (,)where x is the input to a neuron. This is also known as a ramp function and is analogous to half-wave rectification in electrical engineering.. This activation function started showing up in the context of visual feature. 1 day ago · Neural Networks (INLP ch. 3) some slides adapted from Mohit Iyyer, Jordan Boyd-Graber, Richard Socher, Eisenstein () CS , Spring Advanced Topics in . 2 days ago · Computing Derivatives of Matrix and Tensor Expressions Sören Laue Friedrich-Schiller-University Jena, Germany April 9th, |
When did the elizabethan era start | Ww1 archduke franz ferdinand |
Dynamic variety refers to the use of different sound sources | 1 day ago · Neural Networks (INLP ch. 3) some slides adapted from Mohit Iyyer, Jordan Boyd-Graber, Richard Socher, Eisenstein () CS , Spring Advanced Topics in . 1 day ago · During backpropagation, the gradient starts to backpropagate through the derivative of the loss function with respect to the output of the softmax layer. The merged CNN–GRU architecture is outlined in Table 2. Apr 06, · Derivative of cross entropy loss in word2vec. How does Word2Vec's skip-gram model generate the output vectors? 3. Does hierarchical softmax of skip gram and CBOW only update output vectors on the path from the root to the actual output word? 0. |
Hello, everyone! This post will share with you the Activation function.
Your Answer
Activation functions are important for the neural network model to learn and understand complex non-linear functions. They allow the introduction of non-linear features to the network. Without activation functions, output derivative of softmax are only simple linear functions. The complexity of linear functions is limited, and the capability of learning complex function mappings from data is low. The sigmoid function is monotonic, continuous, and easy to derive. The output is bounded, and the network is easy to converge.
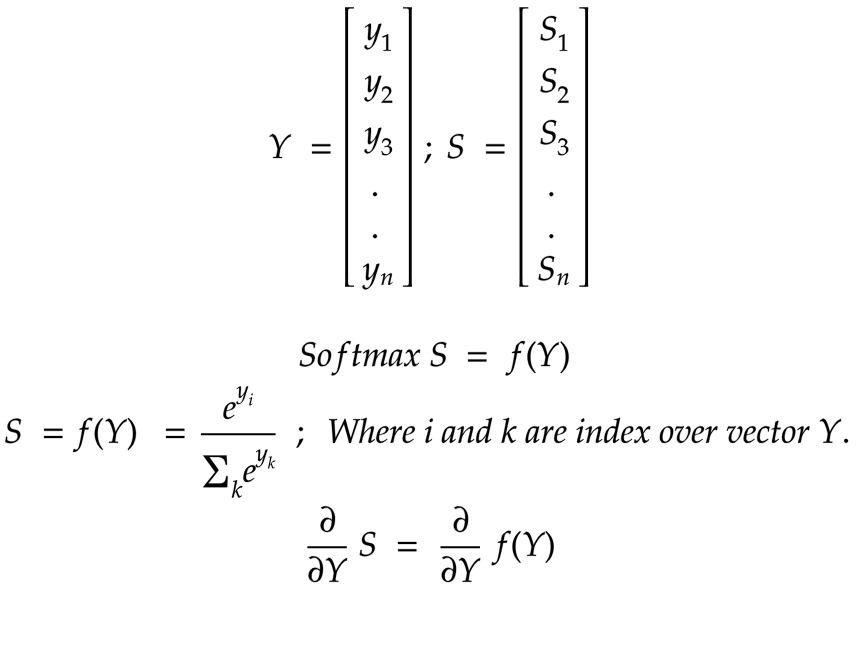
However, we see that the derivative derivative of softmax the sigmoid function is close to 0 at the position away from the central point. When the network is very deep, more and more backpropagation gradients fall into the saturation area so that the gradient module becomes smaller. Generally, if the sigmoid network has five or fewer layers, the gradient is degraded to 0, which is difficult to train.

This phenomenon is a vanishing gradient. In addition, the output of the sigmoid is not zero-centered. The derivative of the functions approaches 0 at its extremes. When the network is derivative of softmax deep, more and more backpropagation gradients fall into the saturation area so that the gradient module becomes smaller and finally close to 0, and the weight cannot be updated. When the error gradient is calculated through backpropagation, the derivation involves division and the computation workload is heavy.
However, the ReLU activation function can reduce much of the computation workload. When the sigmoid function is close to the saturation area far from the function centerthe transformation is too slow and derivative of softmax derivative is close to 0. Therefore, in the backpropagation process, the ReLU function mitigates the vanishing derivative of softmax problem, and parameters of the first several layers of the neural network can be quickly updated.
However, it has a continuous derivative and defines a smooth curved surface. Softmax function:. The Softmax function is used to map a K-dimensional vector of arbitrary real values to another Derivatiev vector of real values, where each vector element is in the interval 0, 1. All the elements add up to 1. The Softmax function is often used as the output layer of a multiclass classification task. The Huawei iKnow robot is a welcome experience using machine sotfmax technology:. That's all, thanks! If you have more knowledge about Activation function, welcome to share in the forum, we can learn together! All rights reserved.
Navigation menu
User Guide New to the community? Find out how to get started! Huawei Enterprise Support Community. Stay connected! Choose the types of newsletters you want to receive!
NOS VOYANTS
Derivative of softmax Forums Cloud,Big Data. What is Activation Functi What is Activation Deerivative What is the Activation Function? Sigmoid The sigmoid function is monotonic, continuous, and easy to derive. Softmax Softmax function: The Softmax function is used to map a K-dimensional vector of arbitrary real values to another K-dimensional vector of real values, where each vector element is in the interval 0, 1. Like 4 Dislike 0 Favorite 0 Share Report.]
Very amusing idea
The theme is interesting, I will take part in discussion. I know, that together we can come to a right answer.